Research institute OpenAI had taught Dactyl, a robotic hand, to solve Rubik’s cube. The company most famously known for its research in artificial intelligence and machine learning, showcased a robotic hand solving a Rubik’s Cube. They have been trying this since 2017.
OpenAI has been trying to train a human-like robotic hand to solve the Rubik’s Cube since May 2017, by using neural networks. Successfully training such a robotic hand to do complex manipulation tasks, lays the foundation for general-purpose robots.
According to Jeff Clune, robotics professor at the University of Wyoming, “What is exciting about this work is that the system learns…It doesn’t memorize one way to solve the problem. It learns.”
Dactyl the robot actually became so good at correcting for perturbations that the researchers had a hard time telling when is part of their system broken; the hand compensated so effectively for disruptive joints and sensors that it was often difficult to figure out that something had gone wrong.
Bringing the power of machine learning techniques to bear on robotics seems likely to make the robots work much better.
Machine information can surpass humans in many different fields.
OpenAI’s approach to solving Rubik’s Cube
The research company trained neural networks to solve the Rubik’s Cube in simulation using reinforcement learning and Kociemba’s algorithm.
Their focus is on the problems that are currently difficult for machines to master: perception and dexterous manipulation.
“We focus on the problems that are currently difficult for machines to master: perception and dexterous manipulation. We therefore train our neural networks to achieve the required face rotations and cube flips as generated by Kociemba’s algorithm.”
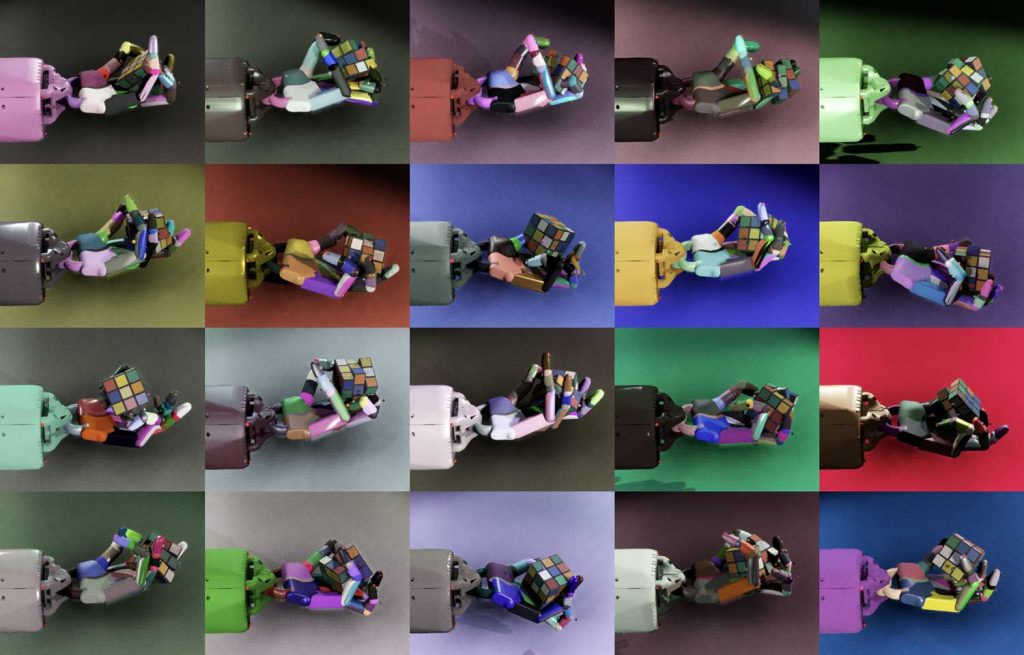
It is difficult to create environments in simulation diverse enough to capture the physics of the real world. Friction, elasticity, dynamics and many other factors are incredibly difficult to quantify and model for objects as complex as Rubik’s Cubes or robotic hands.
OpenAI developed a new method called Automatic Domain Randomization (ADR), which endlessly generates progressively more difficult environments in simulation: their work learns in all environments, then transfers to any newly generated environment.
This eliminates the need for an accurate model of the real world, and enables the transfer of neural networks learned in simulation to be applied to the real world.
The fact that the same technology can be used to approach such different problems shows once more that AI can surpass humans in many different fields.
Article source: Solving Rubik’s Cube with a Robot Hand https://openai.com/blog/solving-rubiks-cube/ https://www.neowin.net/news/openais-robotic-hand-has-taught-itself-the-art-of-solving-the-venerable-rubiks-cube